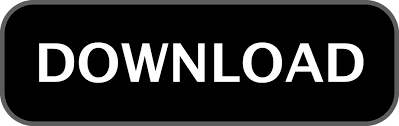
Wu, C.-H., et al.: A real-valued genetic algorithm to optimize the parameters of support vector machine for predicting bankruptcy. In: Oza, N.C., Polikar, R., Kittler, J., Roli, F. 1–16 (2003)ĭuan, K.-B., Keerthi, S.S.: Which is the best multiclass SVM method? An empirical study. Hsu, C.-W., Chang, C.-C., Lin, C.-J.: A practical guide to support vector classification, pp. In: IEEE Second International Symposium on Intelligent Information Technology and Security Informatics (2009) Xia, G., Shao, P.: Factor analysis algorithm with mercer kernel. Sangeetha, R., Kalpana, B.: Performance evaluation of kernels in multiclass support vector machines. In: Proceedings of the 1st Amrita ACM-W Celebration on Women in Computing in India. Sangeetha, R., Kalpana, B.: Optimizing the kernel selection for support vector machines using performance measures. Herbrich, R.: Learning Kernel Classifiers: Theory and Algorithms. Weston, J., Watkins, C.: Multi-class support vector machines, Technical report (1998)īurges, C.J.C.: A tutorial on support vector machines for pattern recognition. Ĭhang, C.-C., Lin, C.-J.: LIBSVM: a library for support vector machines (2001). Sangeetha, R., Kalpana, B.: A comparative study and choice of an appropriate kernel for support vector machines. Schlkopf, B., Smola, A.: Learning with Kernels. (ed.): Support Vector Machines: Theory and Applications, vol. Cambridge University Press, Cambridge (2000) 10(5), 988–999 (1999)Ĭristianini, N., Shawe-Taylor, J.: Introduction to Support Vector Machines.
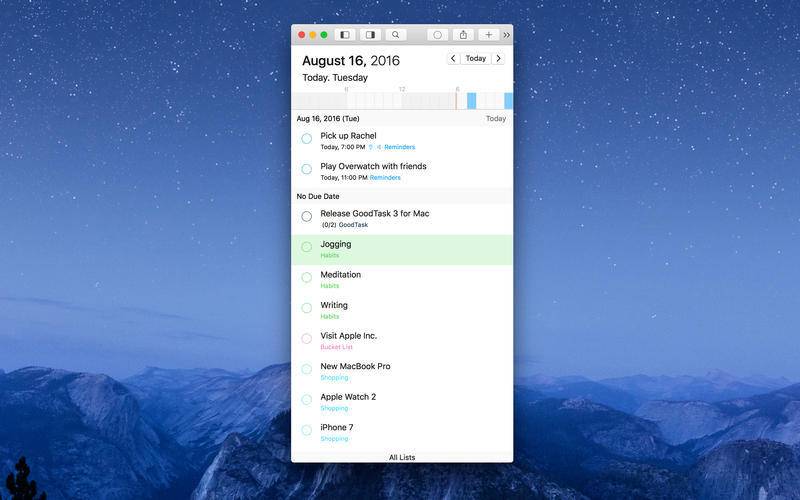
Vapnik, V.: An overview of statistical learning theory. In: 2016 International Conference on Data Science and Engineering (ICDSE), pp. Kumar, R.A., Aslam, M.M., Raj, V.J., Radhakrishnan, T., Kumar, K.S., Manojkumar, T.K.: A statistical analysis of soil fertility of Thrissur district, Kerala. Sehgal, J.L., Mandal, D.K., Mandal, C., Vadivelu, S.: Agro-ecological regions of India, vol. Rani, P.S., Latha, A.: Effect of calcium, magnesium, and boron on nutrient uptake and yield of rice in Kole lands of Kerala. 46(1-3), 131–159 (2002)Ĭrammer, K., Singer, Y.: On the learnability and design of output codes for multiclass problems. 13(2), 415–425 (2002)Ĭhapelle, O., et al.: Choosing multiple parameters for support vector machines. Hsu, C.-W., Lin, C.-J.: A comparison of methods for multiclass support vector machines. Schlkopf, B., et al.: Support vector methods in learning and feature extraction (1998)īurges, C.J.C.: A tutorial on support vector machines for pattern recognition. Finally, a comparative study on the performance is also done for the different choices of the parameters, pointing out their accuracies. Furthermore, different optimization methods like Genetic Algorithm and Particle Swarm Optimization are used to tune the SVM parameters.

The study is conducted for a multiclass soil fertilizer recommendation system for paddy fields. In this study, we propose an improved sigmoid kernel SVM classifier by adjusting the cost and gamma parameters with which a better performance can be achieved. The polynomial kernel seems to be more suitable for performing multiclass SVM classification for the dataset used here. But it is also known that the performance of SVM can be affected by different kernel tricks and regularization parameters like Cost and Gamma. Support Vector Machines (SVM) are advancing rapidly in the field of machine learning due to their enhancing performance in categorization and prediction.
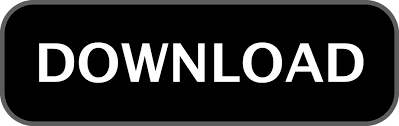